What Is Learning In Facebook Ads
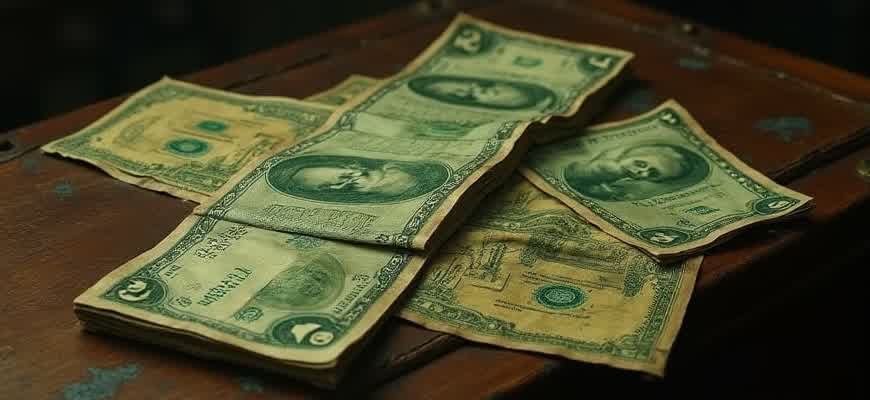
Mastering Facebook Ads involves continuously refining strategies and analyzing data to achieve desired results. Learning in this context refers to the gradual process of understanding how different targeting, ad creatives, and budgets influence campaign performance. The platform uses machine learning to optimize campaigns, and this optimization improves over time as more data is collected.
Key Concept: The Facebook Ads system adapts to performance data, optimizing towards better results as it processes more interactions.
There are several key components involved in learning with Facebook Ads:
- Identifying the target audience
- Creating engaging ad content
- Budgeting and bidding strategies
- Analyzing and adjusting based on performance metrics
Understanding how Facebook Ads work requires recognizing the impact of data-driven decisions:
- Data collection through user interactions
- Continuous ad performance monitoring
- Automated optimization through Facebook's machine learning algorithms
It’s important to note that Facebook's algorithms become more effective over time, meaning that early campaign results may vary from long-term outcomes.
Phase | Action | Outcome |
---|---|---|
Learning Phase | Facebook collects initial data and tests different variables. | Ad performance may be inconsistent, but data begins to shape optimization. |
Optimization Phase | Algorithms use data to adjust bids, placements, and targeting. | Campaigns start delivering more consistent results, improving over time. |
Understanding Facebook’s Dynamic Ad Delivery System
Facebook’s ad delivery system is built to optimize ad performance by selecting the most relevant audience for each ad. It uses a combination of user behavior data, machine learning algorithms, and performance tracking to ensure ads reach individuals who are more likely to engage with them. As a result, advertisers are able to achieve better results with minimal manual intervention, allowing for greater efficiency in ad spending.
The key to Facebook’s dynamic ad delivery is its ability to learn from real-time interactions. The system constantly adjusts the audience targeting, bid strategies, and ad placements to maximize the chances of achieving the campaign’s goals. The following sections will explain how Facebook’s system works and how advertisers can make the most of it.
Key Components of Dynamic Ad Delivery
Facebook's system is based on several core elements that work together to improve ad delivery:
- Learning Phase: The system tests various combinations of ad placements, audience segments, and creatives to determine which performs best.
- Optimization: Once enough data is collected, Facebook optimizes ad delivery to focus on users who are most likely to convert.
- Audience Segmentation: The system continuously refines audience targeting based on behavior patterns and engagement history.
- Budget Allocation: Ads are shown more frequently to audiences that are most likely to drive conversions, based on the budget set by the advertiser.
How Facebook’s Algorithm Learns and Adapts
Facebook’s dynamic delivery system uses machine learning to adapt and improve ad performance. It gathers and analyzes data continuously, which influences future decisions about which ads to show and to whom. This process involves:
- Data Collection: Facebook gathers data from user interactions, such as clicks, shares, and comments, as well as demographic information.
- Model Training: Machine learning models are trained on this data to predict which users are most likely to engage with specific ads.
- Ad Delivery Adjustments: Based on these predictions, the system adjusts targeting, bidding, and placements in real time to improve results.
Optimization Metrics and Performance Tracking
Facebook offers several performance metrics to help advertisers track the effectiveness of their campaigns. These include:
Metric | Description |
---|---|
Click-Through Rate (CTR) | Measures the percentage of people who click on an ad after seeing it. |
Conversion Rate | Indicates the percentage of users who take a desired action after clicking on the ad. |
Return on Ad Spend (ROAS) | Shows how much revenue is generated for every dollar spent on advertising. |
"The goal of Facebook’s dynamic ad delivery system is to find the right balance between targeting the right audience and minimizing wasted ad spend. The system evolves as more data becomes available, leading to increasingly efficient campaigns."
How Facebook Ad Learning Improves Audience Targeting
Facebook's ad learning phase significantly enhances its ability to reach the most relevant users by continuously optimizing targeting. The system collects data from each ad campaign, analyzing user interactions and engagement patterns. Over time, this learning process sharpens Facebook's understanding of who is most likely to respond positively to an ad, thereby improving targeting precision. This leads to better ad performance and reduced wasted ad spend.
The learning phase allows Facebook's algorithm to adjust in real-time based on a variety of factors, such as user demographics, behavior, and preferences. As the system gathers more data, it becomes more adept at predicting which audiences will be most receptive to specific content or offers. This evolving process leads to increasingly effective targeting as campaigns progress.
Key Components of Facebook's Ad Learning Process
- Initial Data Collection: Facebook starts by gathering basic interaction data to identify potential audience segments.
- Optimization for Engagement: Based on the collected data, Facebook optimizes ad delivery for higher engagement rates.
- Audience Refinement: The algorithm continuously fine-tunes its audience targeting as more data is gathered, ensuring more precise ad delivery over time.
"As Facebook learns from user interactions, its ability to predict and target the right audience becomes more accurate, leading to higher conversion rates and improved ROI."
Improvement Over Time
- Initial Reach: The ad targets a broad audience with general characteristics.
- Learning Phase: The system starts to focus on users who show higher engagement levels.
- Refined Targeting: As Facebook collects more data, targeting becomes more precise, reducing irrelevant impressions.
Performance Comparison of Ads
Stage | Reach | Engagement | Cost per Conversion |
---|---|---|---|
Initial | High | Low | High |
Learning Phase | Medium | Medium | Medium |
Refined | Low | High | Low |
Maximizing Conversion Rates Through Facebook Ad Learning
Optimizing Facebook ad performance requires a deep understanding of how the platform learns from user interactions. By leveraging Facebook's machine learning algorithms, advertisers can significantly improve their conversion rates over time. The process involves continuous data collection and analysis, which helps refine targeting, ad creatives, and bidding strategies. Through this approach, Facebook ads evolve to become more effective in reaching the right audience with the right message at the right time.
One key strategy is to take full advantage of Facebook's learning phase. During this period, the algorithm gathers data to optimize ad delivery. Advertisers should aim to provide sufficient budget, clear goals, and effective creatives during this phase to enable the algorithm to perform at its best. Once this learning phase is complete, the system will be able to make highly accurate predictions and deliver ads that yield higher conversion rates.
Key Strategies to Maximize Conversions
- Test and Optimize Creatives: Experiment with different ad formats, visuals, and messages. Use A/B testing to determine what resonates best with your audience.
- Leverage Audience Segmentation: Facebook allows for detailed audience segmentation, enabling advertisers to target users based on interests, behaviors, and demographics.
- Optimize for the Right Event: Instead of just optimizing for clicks, focus on higher-value actions like purchases or sign-ups.
- Set Clear Goals and Budget: Ensure your campaign objectives are aligned with measurable outcomes, and adjust the budget to allow the algorithm to find the best-performing segments.
Maximizing the learning phase's effectiveness requires patience. Avoid frequent changes to the campaign until the system gathers enough data to optimize performance.
Important Considerations
- Tracking Conversions: Use Facebook's pixel to track conversions accurately and feed the algorithm with high-quality data.
- Ad Frequency: Monitor ad frequency to prevent audience fatigue, which can lead to lower conversion rates.
- Retargeting: Create retargeting campaigns to re-engage users who have interacted with your previous ads but did not convert.
Performance Metrics to Monitor
Metric | Description |
---|---|
Click-Through Rate (CTR) | Measures how often people click on your ad after seeing it. |
Conversion Rate | The percentage of users who complete the desired action after clicking your ad. |
Cost Per Acquisition (CPA) | How much it costs to acquire one conversion. |
What Role Does Data Quality Play in Facebook Ad Learning?
In the realm of Facebook advertising, the quality of the data used significantly influences how well an ad campaign performs. When the data fed into Facebook’s learning system is accurate and relevant, the platform is better equipped to optimize ad delivery, identify target audiences, and maximize return on investment (ROI). Poor data, on the other hand, can lead to inaccurate predictions, ineffective targeting, and suboptimal campaign results.
High-quality data allows Facebook's algorithms to better understand user behavior, preferences, and interactions. This understanding is crucial for fine-tuning ad delivery and ensuring that ads reach the right people at the right time. Without clean, reliable data, the platform's machine learning algorithms struggle to learn and improve, which can hinder the effectiveness of campaigns.
Key Aspects of Data Quality in Facebook Ads
- Relevance: Data must reflect the current trends, behaviors, and interests of the target audience.
- Accuracy: Information should be correct and consistent, especially when it comes to demographics and user activity.
- Volume: A larger dataset generally improves the accuracy of machine learning algorithms, but the data must remain relevant and high-quality.
- Timeliness: Fresh, up-to-date data ensures that campaigns reflect current trends and changes in user behavior.
Impact of Low-Quality Data
Low-quality data can cause Facebook’s machine learning to make wrong assumptions about user behavior, leading to wasted ad spend and missed opportunities.
- Inaccurate targeting: Ads may be shown to people who are less likely to engage, reducing the overall effectiveness of the campaign.
- Reduced optimization: The system will struggle to find the right balance between targeting, timing, and content.
- Wasted budget: Without proper data, the algorithm may spend money inefficiently, targeting irrelevant audiences or running ads at suboptimal times.
Summary Table: Data Quality vs. Campaign Performance
Data Quality | Campaign Outcome |
---|---|
High-Quality Data | Improved targeting, higher engagement, and better ROI |
Low-Quality Data | Ineffective targeting, wasted ad spend, poor optimization |
How to Leverage Facebook Ads Learning Phases for Optimal Campaign Performance
Understanding and utilizing the learning phases in Facebook Ads can significantly boost the effectiveness of your campaigns. These phases represent the period during which the system gathers data to optimize your ad delivery. By understanding how these phases work, you can adjust your strategy to ensure better outcomes and faster optimization.
Facebook's algorithm uses machine learning to find the best audience for your ads. During the learning phase, the system analyzes initial interactions and attempts to deliver your ad to the most relevant users. Optimizing this phase is key to improving performance and achieving your marketing goals.
Key Strategies to Optimize the Learning Phase
- Maintain Stable Campaign Settings: Make sure your ad set settings, such as budget and targeting, remain stable. Frequent changes can reset the learning phase and slow down optimization.
- Ensure Sufficient Budget: Provide enough budget to gather meaningful data quickly. If the budget is too small, the system may struggle to find the right audience.
- Give It Time: Allow the learning phase to complete. Interrupting it too soon can prevent Facebook’s algorithm from fully optimizing the campaign.
Common Mistakes to Avoid During the Learning Phase
- Making Frequent Changes: Changing key factors like targeting, budget, or creatives can cause the system to reset the learning phase.
- Underfunding Campaigns: Insufficient budget can prolong the learning phase, making it difficult for the algorithm to reach optimal performance.
- Not Giving Enough Time: It’s essential to allow the system to gather enough data before jumping to conclusions or making changes.
Table: Best Practices and Mistakes to Avoid
Best Practices | Mistakes to Avoid |
---|---|
Keep ad set settings stable | Frequent changes to ad sets |
Allocate a sufficient budget | Underfunding campaigns |
Give enough time for optimization | Interrupting the learning phase prematurely |
Note: Facebook's learning phase typically lasts up to 7 days. If your campaign is new or undergoing major changes, this period may take longer.
Impact of Budgeting Strategies on Facebook Ads Learning Process
When running Facebook ads, the way you allocate your budget can significantly affect how quickly and efficiently the platform learns to optimize your campaign. Budgeting strategies determine how Facebook gathers data and adjusts targeting, bids, and placement over time. The smoother this process, the better your ads perform. Understanding the influence of different budgeting techniques on the learning phase is crucial for maximizing ROI.
Different budgeting strategies can lead to varying outcomes during the learning period. Whether you opt for daily or lifetime budgets, or use campaign budget optimization (CBO), each method impacts how Facebook’s algorithm optimizes for your goals. The right approach can shorten the learning phase, reduce cost-per-action, and deliver more effective results faster.
Types of Budgeting Strategies and Their Effect on Learning
- Daily Budget: A set amount you are willing to spend per day, offering control over daily expenses but can slow down the learning process if too small.
- Lifetime Budget: A total amount for the entire campaign duration, which allows more flexibility and can accelerate learning by allowing Facebook to adjust over time.
- Campaign Budget Optimization (CBO): Facebook automatically distributes your budget across ad sets, optimizing performance. This method can improve overall campaign efficiency and speed up the learning phase.
Key Considerations in Budget Allocation
- Amount of Budget: Larger budgets allow for faster data collection, speeding up the learning process. Small budgets might result in slower optimization.
- Consistency in Budget: Frequent budget changes can reset the learning phase, leading to inefficiencies in ad performance.
- Budget Flexibility: A flexible budget (e.g., lifetime budget) allows Facebook to optimize better by reallocating funds to the best-performing ad sets.
Campaigns with larger and more flexible budgets typically see faster learning and better ad performance. However, consistent and controlled budgeting remains key to maintaining performance stability.
Impact on Learning Phase Timeline
Budget Strategy | Impact on Learning Process |
---|---|
Daily Budget | Slower learning, particularly with low budgets, as Facebook may not receive enough data daily to optimize quickly. |
Lifetime Budget | Faster learning, especially when the budget is allocated over a longer period, providing flexibility for the system to adjust. |
CBO | Quickest optimization and data collection, as Facebook distributes the budget across multiple ad sets based on performance. |
Monitoring and Adjusting Facebook Ads During the Learning Phase
When running Facebook ads, the learning phase is a critical period where the platform's algorithm is optimizing the campaign. During this phase, it's essential to monitor performance closely to ensure the algorithm has enough data to make adjustments. By observing key metrics, you can understand if your ads are on track or if adjustments are necessary. A proactive approach during this phase helps in avoiding potential issues, ensuring the campaign delivers the desired results over time.
It is important to note that excessive changes during the learning phase can disrupt the algorithm’s ability to optimize. However, minor tweaks are necessary to improve performance. Here’s how to approach monitoring and adjusting your ads during this period:
Key Steps for Monitoring and Adjusting Ads
- Track Key Metrics: Focus on metrics such as Cost Per Result, Click-Through Rate (CTR), and Conversion Rate to evaluate ad performance.
- Avoid Frequent Changes: Making too many adjustments can reset the learning phase and delay optimization.
- Use A/B Testing: Implement A/B tests to determine which elements of the ad (such as copy or targeting) work best without significantly affecting the learning process.
Adjustments to Make During the Learning Phase
- Budget Adjustments: Gradually increase the budget if you see positive results to give the algorithm more room for optimization.
- Refine Targeting: Narrow down or expand your target audience based on the data the algorithm has already collected.
- Creative Changes: If CTR is low, consider testing new creatives, but avoid doing it too frequently.
Important: During the learning phase, Facebook's algorithm needs time to gather data. Overloading it with frequent changes can prevent optimal performance.
Key Metrics to Monitor
Metric | What to Monitor |
---|---|
Cost Per Result | Indicates whether the campaign is delivering results within a reasonable cost. |
Click-Through Rate (CTR) | Measures the effectiveness of your ad in prompting engagement. |
Conversion Rate | Shows the percentage of users who completed the desired action after clicking the ad. |