Facebook Conversion Ads Learning Phase
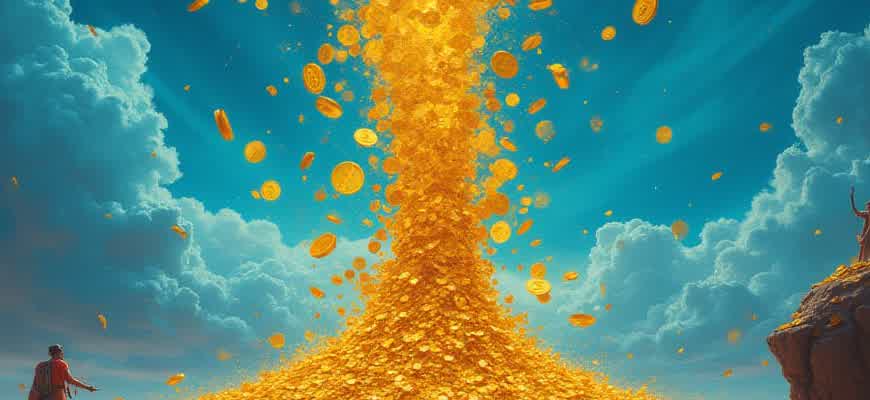
When launching a performance-driven campaign on Meta Ads Manager, it’s crucial to understand how the system collects and processes performance data to improve results. This initial optimization window helps the algorithm determine the best audience and placements for ad delivery.
- The platform requires approximately 50 optimization events per ad set within a 7-day period to complete this stage.
- During this time, delivery may be unstable – expect fluctuations in cost per result and impression volume.
- Frequent edits or budget changes reset the system’s progress, delaying efficient delivery.
Note: The faster the ad set reaches the required number of events, the sooner it exits the learning state and achieves stable performance.
To monitor progress, advertisers can review the delivery status and performance metrics directly in the Ads Manager dashboard. Below is a quick breakdown of key indicators during this period:
Metric | Description | Implication |
---|---|---|
Learning | Ad set is still being optimized | Performance not yet stable |
Learning Limited | Insufficient optimization events | Consider adjusting targeting or budget |
Active | Optimization completed | Ad set is performing consistently |
How the Learning Phase Impacts Ad Delivery
When a new campaign or ad set is launched, Facebook's system begins gathering performance data to optimize who sees the ads. During this calibration period, delivery can be unstable, with results fluctuating significantly. This happens because the system is still testing different audience segments, placements, and times to identify the most responsive combinations.
Insufficient conversion data during this phase can lead to suboptimal distribution. If the system doesn't gather enough meaningful interactions (typically around 50 conversions per ad set per week), it may struggle to exit the exploration period, causing underperformance.
Key Effects of the Learning Period
- Volatile Performance: Results often vary as the algorithm experiments with delivery.
- Higher Costs: Without stable optimization, cost per action may temporarily rise.
- Delayed Scaling: Campaigns might not reach full potential until this phase ends.
Ads will not achieve consistent results until the system gathers sufficient conversion data to optimize delivery effectively.
- Launch ad set
- System collects conversion events
- Patterns identified and delivery refined
- Exit learning phase and stabilize performance
Phase | Conversions Required | Optimization Status |
---|---|---|
Learning | < 50/week | Unstable |
Post-Learning | ≥ 50/week | Optimized |
Triggers That Reset the Learning Phase
When running conversion-focused campaigns on Facebook, the system uses a learning process to optimize ad delivery. This phase helps the algorithm understand the ideal audience and actions. However, specific changes can interrupt this learning process, forcing it to restart.
Restarting the learning process can temporarily decrease performance, so it's important to recognize what triggers this reset. Below are the key actions that can cause Facebook's delivery system to re-enter the optimization phase.
Key Modifications That Cause a Learning Reset
Important: Any significant edit that alters the way your ad set is delivered or evaluated will result in the optimization system re-learning.
- Changing the event Facebook is optimizing for (e.g., switching from "Add to Cart" to "Purchase").
- Making major adjustments to the daily or lifetime budget (typically 20%+).
- Modifying the target audience, such as interests, demographics, or custom audiences.
- Altering bid strategy or switching between automated and manual bidding.
- Editing creatives, including primary text, headline, or media files.
- Audience Tweaks: Narrowing or broadening your targeting range resets the delivery pattern.
- Conversion Goal Change: Adjusting the conversion goal signals a new optimization direction.
- Large Budget Shift: Sudden increases or decreases in budget disrupt performance data continuity.
Action | Effect on Learning |
---|---|
Change in Optimization Event | Full learning phase reset |
Significant Budget Change | Triggers re-learning |
Audience Redefinition | Restarts learning |
Creative Update | New performance data required |
Minimum Required Conversions to Exit the Learning Stage
In Facebook's ad delivery system, a campaign enters a trial phase during which the algorithm collects performance data to optimize future results. Exiting this period requires a specific number of confirmed conversion actions, without which optimization remains limited.
Campaigns that do not meet the required action volume remain unstable and inefficient, often resulting in fluctuating cost-per-result and inconsistent delivery. To stabilize performance, it's crucial to hit the minimum action goal within a set timeframe.
Key Metrics for Optimization
Note: To ensure stable delivery and accurate performance prediction, the algorithm needs a consistent flow of at least 50 conversion events per ad set per week.
- Conversion Events: These include purchases, leads, form submissions, etc.
- Per Ad Set Basis: The threshold applies to each ad set, not the entire campaign.
- 7-Day Rolling Window: Conversions are counted over a seven-day period.
- Launch the ad set with a clear and specific conversion goal.
- Monitor the conversion count daily to ensure alignment with the threshold.
- Adjust targeting, budget, or creatives if performance stalls below the minimum.
Element | Requirement |
---|---|
Conversions Needed | Minimum 50 per week |
Timeframe | Rolling 7 days |
Level of Measurement | Per ad set |
Budget Allocation Strategies During Learning
When initiating a new conversion-focused campaign, how you distribute your budget in the early stages directly influences the algorithm’s ability to collect performance data. Insufficient or uneven budget allocation often leads to delayed optimization, extended learning time, or inefficient ad delivery.
To achieve faster stabilization and maximize return on investment, advertisers should focus on maintaining consistent daily spend and balancing distribution across ad sets. This enables Facebook’s delivery system to gather enough conversion signals for effective optimization.
Key Budgeting Techniques
- Minimum Daily Budget Rule: Ensure each ad set receives enough budget to generate at least 50 conversions per week.
- Gradual Scaling: Increase budget by no more than 20–30% per adjustment to avoid resetting the learning period.
- Consolidation: Reduce the number of ad sets to prevent audience fragmentation and improve signal strength.
Tip: If an ad set shows strong performance signals but lacks budget, reallocate from underperforming sets to accelerate learning without full reset.
- Start with even distribution across ad sets targeting distinct audience segments.
- Monitor Cost per Result and Conversion Volume daily.
- Adjust budget towards high-performing sets based on real data after 3–4 days.
Ad Set | Initial Daily Budget | Adjustment After Learning |
---|---|---|
Audience A | $50 | $65 (based on high CTR) |
Audience B | $50 | $35 (based on low conversions) |
Adjusting Targeting Without Restarting Learning
When refining audience parameters within a conversion-focused campaign, it's crucial to avoid actions that trigger a reset of the system’s optimization process. Minor changes allow you to refine who sees your ads without forcing the platform to relearn performance patterns from scratch.
Focus on granular adjustments that maintain campaign stability. Instead of swapping audience sets entirely, use expansion tactics or modify existing parameters incrementally.
Recommended Methods
- Use Audience Expansion to broaden delivery without replacing the original group.
- Adjust age range or gender slightly rather than overhauling them.
- Exclude underperforming interest layers instead of rebuilding the full targeting structure.
Avoid duplicating ad sets to test new audiences – this often leads to fragmented data and extended optimization periods.
- Navigate to your current ad set.
- Edit targeting with minimal shifts (e.g., adding one interest at a time).
- Monitor performance trends for at least 3–5 days before making additional changes.
Action | Impact on Optimization |
---|---|
Change one interest | No reset |
Swap entire audience | Resets learning |
Use Lookalike Expansion | Preserves current optimization |
Monitoring Performance Metrics Within Learning
During the initial phase when an ad set is still optimizing, it's critical to closely observe specific indicators that reflect whether the system is effectively collecting conversion data. These metrics serve as signals of progress and can indicate whether adjustments are necessary before the budget is exhausted.
Focusing on the right performance signals early helps avoid misinterpreting fluctuations that are normal during data accumulation. Instead of relying on overall campaign performance, track ad set-level indicators that provide a clearer picture of algorithmic stabilization.
Key Metrics to Track
- Cost per Result (CPR): Observe trends rather than isolated spikes. A declining CPR may indicate stabilization.
- Impression-to-Conversion Ratio: Useful to assess if the creative and targeting are aligning well.
- Click-Through Rate (CTR): Helps evaluate ad engagement while conversion data is still limited.
If an ad set fails to reach approximately 50 conversion events within 7 days, performance optimization remains incomplete and future results may stay inconsistent.
- Check daily conversion counts against your optimization goal.
- Compare frequency and CTR across creatives to identify ad fatigue or mismatch.
- Evaluate landing page load time, as delays can disrupt conversion tracking.
Metric | Target Indicator | Action if Underperforming |
---|---|---|
Cost per Result | Decreasing over time | Review targeting and placement if rising |
Conversions | At least 50 in 7 days | Increase budget or broaden audience |
CTR | >1% | Test new creatives if below |
Common Errors That Extend the Facebook Conversion Ads Learning Period
In Facebook Ads, the learning phase is a crucial period for the algorithm to optimize ad delivery. However, certain mistakes can extend this phase, leading to inefficiencies and delayed results. Understanding these mistakes can help advertisers better manage their campaigns and minimize unnecessary delays in reaching optimal performance.
When the learning phase is prolonged, Facebook's algorithm struggles to gather enough data to properly optimize for conversions. These issues can arise from a variety of factors, including improper targeting, lack of sufficient budget, or inconsistent creative strategies. Below are the most common errors to avoid to ensure a faster transition from the learning phase to stable performance.
1. Inadequate Budget Allocation
One of the primary reasons campaigns linger in the learning phase is setting an insufficient budget. Without enough funds, Facebook cannot gather enough data points to effectively optimize the ad delivery. Here’s why:
- Low daily budget: Limits Facebook’s ability to accumulate the necessary data to make informed decisions.
- Frequent budget changes: This resets the learning phase, forcing the algorithm to start over.
Tip: Set a budget that is large enough to gather 50 conversions per week for each ad set to help the algorithm optimize efficiently.
2. Poor Audience Targeting
Another frequent mistake is narrowing the audience too much. A smaller audience can make it harder for Facebook's algorithm to find enough people who are likely to convert. This leads to slower learning as the algorithm doesn’t have enough data to optimize delivery.
- Overly specific targeting: Limiting to a very narrow audience might restrict the pool of potential conversions.
- Not testing different audiences: Sticking to a single audience set without testing others can prevent the algorithm from finding the most responsive group.
3. Inconsistent Ad Creative
Changing your ad creatives too frequently can reset the learning phase. Facebook requires consistency in both creative and copy to accurately track the effectiveness of the ads. This can significantly prolong the learning period.
- Frequent changes in ad design: A new creative too soon may cause the algorithm to start the optimization process over.
- Not maintaining consistent messaging: Changing the ad copy too often can confuse the algorithm and disrupt performance stability.
4. Unstable Conversion Events
Using multiple conversion events or switching between them during the campaign can interfere with Facebook’s learning process. The algorithm needs a consistent signal to optimize effectively.
Important: Focus on a single conversion event throughout the campaign to ensure the algorithm is receiving clear signals.
5. Low Conversion Volume
If your campaign doesn’t generate enough conversions to meet the minimum threshold (usually 50 conversions per week), the algorithm will struggle to optimize efficiently. This leads to a prolonged learning phase or poor performance overall.
- Ensure the target audience size is large enough to generate sufficient conversions.
- Test with different campaigns or ad sets to increase the volume of conversions.
Common Mistake | Impact on Learning Phase | Recommended Action |
---|---|---|
Inadequate Budget | Limits data accumulation and optimization | Increase budget and avoid frequent changes |
Poor Audience Targeting | Reduces the pool of potential conversions | Test broader or varied audiences |
Inconsistent Ad Creative | Resets the learning process | Maintain consistency in ads |
Unstable Conversion Events | Confuses algorithm optimization | Use a single consistent conversion event |
Low Conversion Volume | Prevents proper optimization | Increase conversions through larger audience or ad sets |
When and How to Scale After Learning Completion
Scaling your campaigns after the Facebook ad learning phase is crucial for maximizing results. However, it is important to wait for the learning phase to complete before making any significant changes. This phase ensures that Facebook’s algorithm has gathered enough data to optimize performance effectively. Once the learning phase has ended, you can confidently scale your campaign, but the approach should be measured and strategic.
Understanding when to scale involves monitoring key metrics such as Cost per Acquisition (CPA), Return on Ad Spend (ROAS), and overall campaign stability. Once your campaign stabilizes with consistent results and a sufficient number of conversions, scaling can be initiated. It's important to increase budgets or expand targeting gradually to avoid disrupting the algorithm's optimization.
Steps to Scale After Learning Completion
- Increase Budget Gradually: Avoid large jumps in budget; increase it by 10-20% at a time to allow Facebook's algorithm to adjust.
- Expand Targeting: Broaden your audience or use additional lookalike audiences to increase reach.
- Test New Creative: Refresh your creatives periodically to avoid ad fatigue and maintain audience engagement.
- Monitor Key Metrics: Track performance indicators like CPA, ROAS, and frequency to ensure scaling doesn’t lead to inefficiency.
Scaling too quickly can lead to the loss of optimization gains made during the learning phase. Gradual and controlled scaling ensures that Facebook’s algorithm continues to optimize efficiently.
Key Considerations for Scaling
- Timing: Scaling should only occur once you have a sufficient number of conversions and stable metrics post-learning phase.
- Maintain Efficiency: Always check if scaling affects your CPA negatively; if so, scale back to maintain profitability.
- Incremental Changes: Ensure gradual increases in budget and audience size to minimize any potential disruption to ad performance.
Scaling Methods
Method | Description |
---|---|
Budget Increase | Increase daily budget by 10-20% to maintain algorithm performance while boosting reach. |
Audience Expansion | Expand your audience base using broader targeting or new lookalike audiences. |
Creative Refresh | Introduce new creatives to keep the campaign fresh and avoid audience fatigue. |